Panel Discussion: The Future of AI in Product Design
Augmented simulation features inside computer-aided engineering (CAE) tools are accelerating the design decision process with machine learning. The power of machine learning (ML)-based artificial intelligence (AI)-powered design combined with physics-based simulation-driven design leveraging the latest in high-performance computing (HPC) is just being realized. While predictive data analytics techniques long associated with business-centric data are being aggressively deployed on asset-centric data to enhance manufacturing, warranty, and testing performance.
At Altair’s recent Future.AI event, a 35-minute panel discussion took place exploring the role AI will play in the future of product design. Moderated by Dr. Carsten Bange, founder and CEO of Business Application Research Center (BARC) GmbH, the panel includes Altair experts and technologists Dr. Fatma Kocer, vice president of engineering data science, Anthony McLoughlin, vice president of data analytics sales, Christian Kehrer, system modeling business development manager, and Marco Fliesser, EMEA technical director of data analytics.
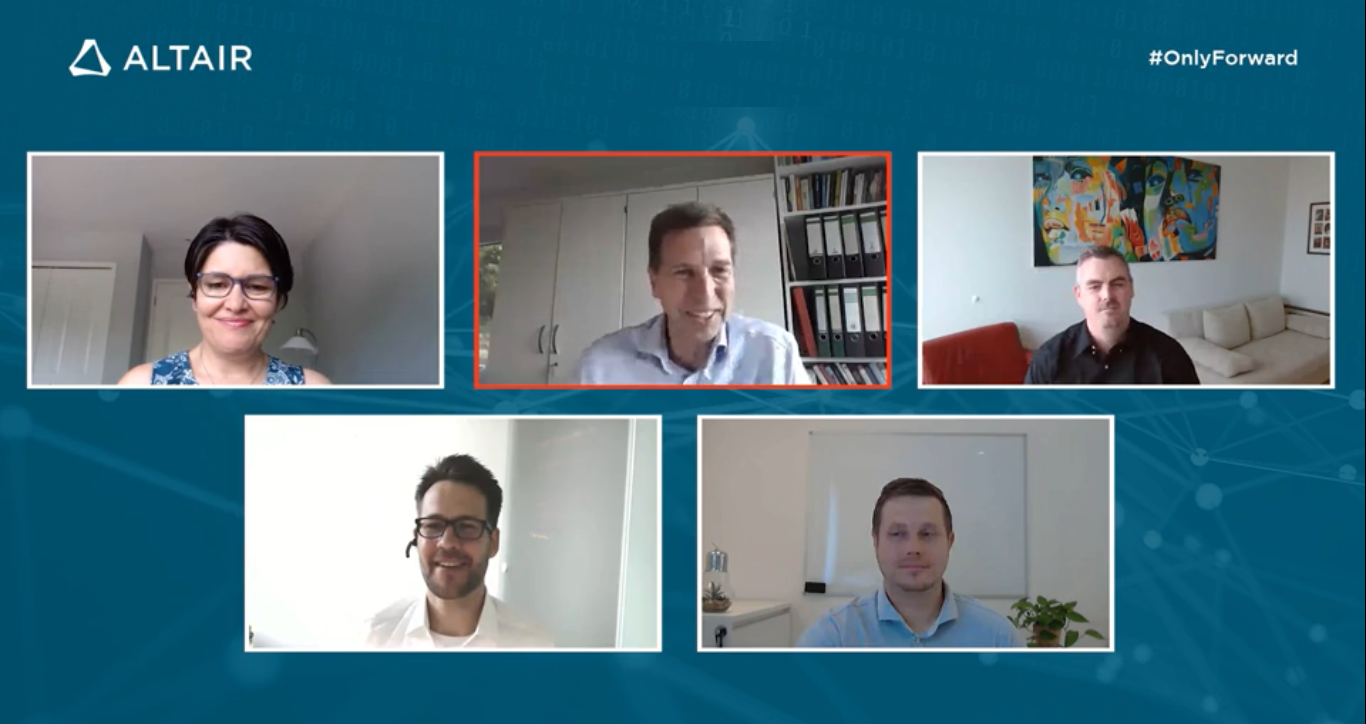
Below are some of the key questions asked during the panel discussion:
What are the key areas of engineering data science?
Anthony McLoughlin: There are two key areas that we’re at focusing on. Number one is inside of CAE or product development, that’s about automation of workflows, being able to build and simulate models faster. And secondly, being able to discover new and better designs faster. Outside of CAE but still very engineering-driven, is the existing data use cases such as warranty analytics, being able to leverage data and engineering knowledge to reduce the number of recalls for a given product, smart factory, predictive maintenance, quality analytics, these sorts of things. So those existing data use cases outside of engineering but engineering driven. And of course, new use cases outside of CAE.
In the area of testing for example, there is vast amounts of data and potential to automate and reduce the amount of physical testing. All of these use cases are focus areas, whether it be in CAE, the engineering department, the quality department, testing, or manufacturing. They’re all very driven by engineering domain knowledge. Implementing the use cases I just mentioned are absolutely game changing for a manufacturer. But the real key problem is that there’s a staffing of domain knowledge gap and engineers are really the perfect fit for it in my view. And that’s why we believe at Altair that engineers will know the potential of AI inside and outside of CAE. Our focus at Altair is to empower engineers, to help them make that journey to data science, and to do it faster.
We already talked about how we want to deploy these ML-based solutions. Where do you see the big differences compared to a physics-based approach when you talk about design decision making?
Fatma Kocer: As engineers we create a lot of data but we haven’t really been data disciplined and there are reasons for that. Our data is usually very large files, we can’t always store them, and once you find a good design you sort of move on and delete everything else because you have to open room for the new incoming data. That ties into why an ML data-driven approach hasn’t picked up as fast as it did in other industries. In an ML-based solution, we deduct performance of a specific application from a data set and learn from the data. As a result, we need data upfront but we don’t have to make assumptions about the physics, the material properties, the load cases, etc. Once we’ve trained an ML model, the inference for a new design is really fast and that allows us to do wide design exploration and come up with more innovative designs.
With a physics-based approach, we induct from what we already know of the physics, the materials, and the load cases. But we have to make assumptions to simplify the problem because otherwise they will require even longer computational times using very high performing computers. Physics-based models don’t require data upfront, but they require implementing the physics that you know, simplifying, and lengthy preparation times to convert a design to a CAE model such as meshing, applying loads, and boundary conditions.
One other topic where ML models shine is with even more complex devices that companies are designing and manufacturing. Sometimes you don’t even know how to simulate the physics, but given enough data, we can still create predictive models to predict performance or at least understand the relationship between the design changes and the performance. That can speed up our learning about an application that we don’t quite know the governing rules of.
What is Altair® DesignAI™ and what does it do?
Fatma Kocer: Available in Altair One, DesignAI is an application that automates attribute extraction, data augmentation, job submission, machine learning, and design optimization. To me, DesignAI adds power to existing simulations by extracting data from simulations and knowledge from data. Engineers can use this knowledge to make better design decisions. At Altair, we do not expect our users to be data scientists or be able to leverage data science, so DesignAI automates data prep, auto ML, and deployment with optimization algorithms. We also do not expect our users to blindly believe and use black box ML models. In Altair solutions, you’ll see there is automation, ML, and explainable AI.
DesignAI shows the users which attributes are contributing to the changes in the design performance. We enable engineers to interact with the data so that they can do their own investigations as well as their own design explorations. As we are exploring a merge of technologies, we’re also exploring the merge of data from different sources such as simulations, test, manufacturing, operations, and how they can be used to complement each other. DesignAI is an environment that will eventually enable us to use test and operational data.
All that you’ve mentioned sounds really impressive. But what about the smaller companies, the SMBs? Do they actually have the funding, the expertise to support this?
Christian Kehrer: What large organizations and SMBs do have in common is that they have a fair amount of data and they have the detailed knowledge of their products. These are two main requirements to benefit from AI, ML, and data science. What’s more relevant for SMBs is that Altair has the expertise to work on these specific projects for customers of any size. It means we can do the proof of concept, transfer to know-how, and help them understand the approaches to benefit from their data. On the other hand, SMBs can help us understand the technical background and the business behind the data. We can really help SMBs accelerate the ramp-up phase without the need for hundreds of data scientists or technical experts.
We are moving more and more to cloud-based solutions, so being able to have these approaches not only as a premise but while keeping administrative efforts as low as possible and providing these solutions on demand has a bigger impact for SMBs. The interest to want these technologies is nearly at the same level as large organizations.
To watch the full conversation where more questions about the future of AI in product design are discussed, check out the panel discussion here.