Generative AI Security: View from the CISO’s Office
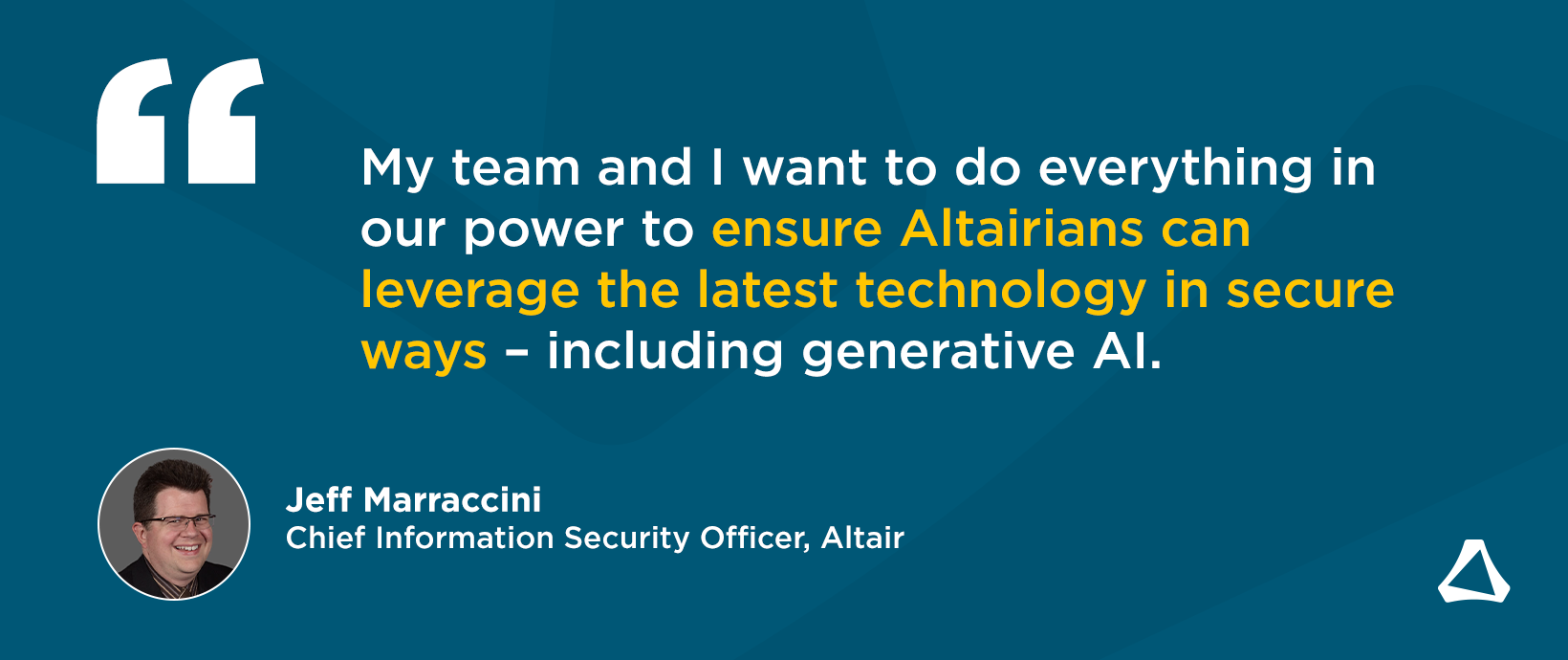
I know I’m stating the obvious, but generative artificial intelligence (AI) is all the rage. It has attracted attention from nearly everyone: businesses, governments, media, and the public alike. Undoubtedly, it’s a supremely intriguing technology, one that likely signals some major changes in the ways in which we live, work, and play. It’s also a very complex topic, especially from a security standpoint.
As a chief information security officer (CISO) for a leading global technology company, I’m always analyzing the benefits and drawbacks of these kinds of exciting, yet still emerging innovations, and trying to understand how our employees – as well as my team – can safely maximize the power of these technologies. And although “security” may be in my title, I don’t approach each day gripped with a sense of trepidation and anxiety over potential security incidents and vulnerabilities. In fact, I often think about my role in terms of empowerment; as in, how can I empower Altairians and our customers with great tools and technologies in safe, sustainable ways? In other words, a CISO’s role is to maintain an equal balance of positive freedom (“freedom to”) and negative freedom (“freedom from”). Everyone within our security ecosystem and I strive to help Altairians and our customers leverage every technology they possibly can possibly can so we can maintain our cutting edge.
Generative AI certainly has the potential to be one of those transformational technologies for our team and our customers – but it doesn’t come without security risks. And while generative AI security isn’t the topic’s flashiest side, it’s certainly one of the most important.
Large Language Models (LLMs): Making Generative AI Tick
I won’t be walking through the detailed inner workings of generative AI; you can find simple, informative guides elsewhere. But a basic understanding of how generative AI works will help us begin. The bedrock of generative AI and machine learning processes is training data. Many popular generative AI tools are trained using large language models (LLMs), which are artificial neural networks capable of processing vast amounts of data. Much of this data is scraped from the internet.
In general, when I look at LLMs, there are really two major categorizations I use: those that are “public” and those that are meant for more “enterprise-level” use. This is a basic picture, but it serves our objectives. Generative AI tools trained via public LLMs can be accessed via web browsers or simple applications. These tools can do a lot of great things in text, images, audio, etc. Enterprise LLMs add another layer on top of this data that specializes the generative AI tool for a specific organization. An example of this type of solution is Microsoft 365 Copilot; Google and OpenAI also have similar solutions. There are also some open-source LLMs that can connect with Microsoft OneDrive or document repositories that could also be classified as enterprise LLMs.
LLMs and Generative AI: General Security Considerations
LLMs are imperfect creations. Their inherent structure means there are various concerns from a security standpoint. Of course, there are also various legal and ethical concerns that come with generative AI, but I’ll let others cover that topic. Let’s explore some of the main generative AI security concerns that any organization must consider.
The first and most pressing concern for many organizations thinking of deploying or experimenting with generative AI tools revolves, unsurprisingly, around data security. It’s incomprehensible how much data an LLM can comb through. Naturally, people and organizations want to ensure their sensitive data doesn’t inadvertently end up as fodder for unauthorized LLMs and generative AI solutions. Some of the main questions that (should) arise when thinking about data security as it relates to generative AI should be:
- Where is my data going and how is it being used?
- How long will my data stick around?
- Will my data be used to train subsequent generations of LLMs/generative AI tools?
- How can I ensure my data isn’t susceptible to unwanted trawling?
Answers to these questions are increasingly being covered by open-source projects such as eSentire's recently announced eSentire LLM Gateway. Current web browsers also include profiles that allow individuals and organizations to "wall off" personal use of the web from organizational use, and it’s possible to set up a separate organizational profile when using generative AI in browsers to prevent accidental use of content from previous web sessions.
The next most pressing concern is generative AI’s tendency to hallucinate. AI “hallucinations” refer to confident responses by an AI that are not justified by its training data. Hallucinations aren’t unique to AI. Humans have been “hallucinating” since the dawn of time; it’s often called “making a mistake” or simply “being wrong.” What makes AI hallucination so tricky is that AI tools can be very convincing and invent seemingly extensive logical justifications for its output that are essentially made up. Hallucinations are especially dangerous when AI is involved in the security ecosystem. For example, generative AI-powered alerts and security reports can misidentify serious security issues (false positives) or miss them altogether. In addition, bad actors can also exploit these tools to create convincing alerts and reports. Using a powerful text-based generative AI tool, a bad actor could send out a mass phishing email or internal security report in my tone of voice to convince other team members to take desired actions.
There are other security concerns as well. Current generative AI tools aren’t as “explainable” as many other legacy AI and machine learning tools, meaning it can be hard to pinpoint what data an AI used how to arrive at a given output. Many times, LLMs crawl data that’s incorrectly attributed or not attributed at all; if I were to write somewhere that “All the world's a stage, and all the men and women merely players” without giving Shakespeare his proper attribution, an LLM may think I came up with that storied line rather than the legendary English playwright who died more than 400 years ago. Such problems with attribution, when scaled up to the size of powerful LLMs, can be far more troublesome and raise a host of technological and legal issues.
The Generative AI Security Landscape and Altair’s Approach
Zooming out to today’s generative AI security landscape, companies have taken a variety of approaches concerning the use of generative AI within their ranks. Some, like JPMorgan Chase, have completely blocked access to popular generative AI tools like ChatGPT for fear of data leaks. I can totally understand this approach. Generative AI isn’t a tool where it’s wise for large organizations to “use first and ask questions later.” People need to build trust in these technologies, and that will take time.
At Altair, we’ve opted to let Altairians use generative AI, and have issued careful, detailed guidance as to how to use these technologies in secure ways that won’t jeopardize our data or our customers’ data. As I mentioned, I view my role through the lens of empowerment; my team and I want to do everything in our power to ensure Altairians can leverage the latest technology.
We monitor the generative AI security landscape 24/7, always staying up to date with the latest industry best practices. As a CISO, I know that emerging technologies – especially those like generative AI – will always carry some element of risk. Industry-leading technology companies like Altair do not, and have never, existed in a 100% secure landscape – hardly anyone does. Modern technology is supremely complex and features more moving parts every day, it seems. That said, my team and I are confident in our guidance and our approach.
Conclusion
As generative AI technology matures, security best practices will inevitably change. From the inner workings of the technology itself to external rules and regulations on its use and scope, many factors will affect how organizations can keep users secure while empowering them to leverage the technology’s full power. My team and I will always be ahead of the curve when it comes to generative AI security (and beyond, of course). Already we’re doing some magnificent things that allow users to utilize and tie in generative AI capabilities within our existing solutions in safe, secure ways.
In generative AI, as in all things, we must exercise caution and care. We must implement these tools in considered ways. Doing so will allow us and our customers to do marvelous things not just now, but in the future as well.