Credit Risk Analytics and Machine Learning in the Financial Industry
In the era of the new digital economy, financial services executives are looking for ways to distinguish their organizations, remain relevant to customers, find new markets that will drive growth and revenue, and leverage technologies that increase margins and reduce risks.
With so much data available to them, financial institutions that take advantage of data science, data analytics, predictive analytics, and machine learning can empower people to make better decisions so they can meet business objectives. Accurately predicting future consumer behavior allows credit risk analysts, financial marketing analysts, and fraud detection teams to better deploy strategies that both capitalize on opportunities and act as preventative measures against disruptive forces to their business models.
Altair’s data analytics and credit risk analytics solutions provide market-leading data analysis, credit risk analysis, and financial analysis tools that empower people to make smarter, more informed decisions. Our financial software solutions combine common data science methodologies and sophisticated statistical algorithms with an interface that business analysts and data scientists can use to find insights about their consumers and strategies. These insights can lead to new opportunities, increase loyalty, and reduce risk that could impact both the bottom line and brand equity.
What is Data Science?
Data science is an interdisciplinary field that uses scientific methods, statistical algorithms, and computational systems to find insight from data. Typically, this data comes from disparate sources, is voluminous, and often requires preparation and manipulation before teams can begin the data analysis process. The goal of data analytics is to find patterns of behavior in historical data from which to draw conclusions and predict future trends and behavior. Organizations can truly realize the power of data analytics, credit risk analytics, and predictive analytics when algorithms can adjust to new data patterns and refine analyses without relying on human intervention.
What is Machine Learning and Predictive Analytics?
Machine learning is a subset of predictive analytics and is foundational to the development of robotics that cognitively interpret, understand, learn, perform and adapt in real-time when executing analytical routines against data that’s constantly changing. In the context of predicting consumer behavior with regards to credit risk analytics or responding to a marketing campaign, machine learning is defined by algorithms and systems that improve themselves without relying on explicit programming to adapt to changing patterns and recommend appropriate actions.
Machine learning learns from observing millions, or even trillions of data points. Common use cases in financial services include anticipating consumer financial goals, and providing smart recommendations to consumers as they complete transactions on their mobile devices.
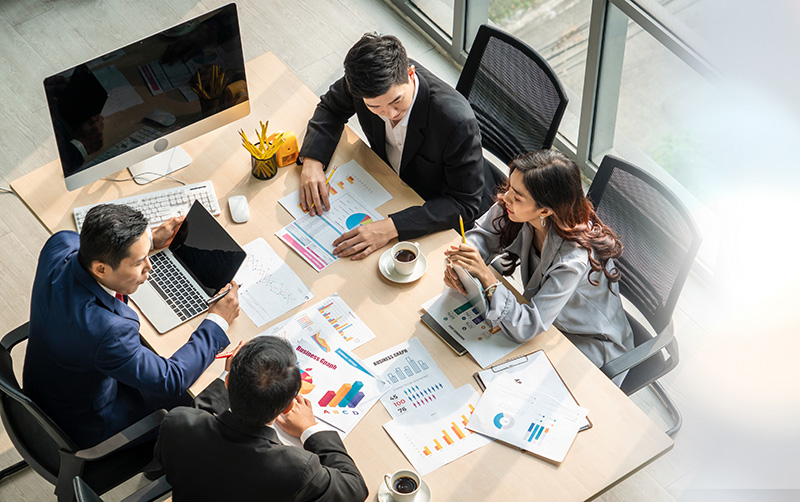
Altair credit risk analytics solutions bring people and teams together in strategy alignment.
Predictive analytics and machine learning both endeavor to reach the same end: to provide insight from the hundreds or thousands of dimensions people can’t see. Predictive analytics relies on a person’s ability to work out and test the relationships between cause and effect. This is accomplished by refreshing the data in the models being used, or manually updating the model design itself. Machine learning removes the person from this step by recalibrating models after their initial design.
Machine Learning: Artificial Neural Network
A subset of machine learning is a concept known as deep learning, or multi-layer neural networks. Artificial neural networks (ANN) adapt and learn from vast amounts of data. Relatively new to statistics, data mining, and credit risk analytics, ANN are based on human brain functionality and attempt to emulate the process by which we people make decisions. Neural networks emulate the human brain to perform nonlinear deductions. Neural network systems produce better credit risk analytics decisions by learning from previous interactions or transactions to formulate conclusions.
The Importance of Financial Risk Analytics and Data Science
What makes data analytics and financial risk analytics so important today? To start, businesses retain more data than ever before. Getting insight out of terabytes or petabytes of data to make actionable decisions is data science teams’ job. Empowering better decision-making, identifying new opportunities, and making decisions based on trusted, quantifiable data are the byproducts of data science teams using various statistical tools and analytical methodologies.
Data science models can develop outputs from large datasets in real or near real time. These outputs help predict what will happen in the future; model algorithms are sophisticated enough to adapt to fluctuations in data as consumer behavior patterns change. Data science has become an essential component to how businesses go to market with new products and services, and how businesses control costs and craft credit risk analytics strategies.
Accelerating cost efficiencies and developing faster time-to-market strategies requires professionals to rethink process automation, workforce mobility, and supply chain efficiencies. Growing revenue and increasing loyalty requires optimized messaging and marketing campaigns, real-time information, and personalized consumer engagement. In the digital economy, data-centric services and universal data access will help businesses unlock new revenue streams and reach new markets.
Today's Financial Analytics Capabilities
The power of data analysis in the financial industry is immense – especially in the credit risk industry. Today’s solutions are incredibly powerful and can optimize credit risk analytics strategies, collection strategies, fraud risk mitigation, and more. So how do they work?
Propensity Modeling and Scoring for Collections
Propensity modeling is a statistical approach and series of techniques used by data scientists to estimate the likelihood of consumers to act on certain behaviors, like the propensity to buy a new financial product or service or to repay outstanding line of credit debt. Essentially, different probabilities are assigned to consumers based on shared features (i.e. income range or age groups) to create accurate predictions of future behavior. Predictive analytics work well with multi-dimensional data and enable financial organizations to create propensity scoring models to better understand how their consumers will respond to tailored messaging campaigns.
Want to learn how to use machine-learning powered scorecards to bolster your credit, scoring, and risk strategies? Watch this on-demand webinar, “Support Lending Decisions and Minimize Risk Using Scorecards.”
Credit Risk and Fraud Detection Analysis
Traditional rules-based systems are ineffective as soon as fraudsters change tactics. Simply adding more rules is inefficient; it’s difficult to detect anomalous behavior in large transactional datasets, especially when there are ever-evolving fraud tactics.
Fraud detection and prevention is about connecting multiple data points to discover potential fraud before it happens. Using predictive analytics allows financial service organizations to discover events that are anomalous when compared to commonly observed behavior. Finding interactions between devices, products, locations and other data points, and aggregating relating data points to the entity level (the customer, the employee, and/or the business) connects substantial quantities of knowledge with all people who have previously interacted with that knowledge.

Cutting-edge credit and fraud risk solutions are more important than ever.
Financial companies struggle to find balance between maximizing revenue when offering a line of credit to an applicant and minimizing the propensity of the applicant to default on payment. Combining disparate datasets with large amounts of transactional data, bank account/product held data, and demographic data to create an accurate credit risk scoring model is difficult. Predictive analytics helps financial organizations better segment product holders and create scoring models that identify new opportunities, offering increased limits while minimizing non-payment losses. Such scoring models can be built out of internal data and combined with external data sources, such as data that comes from risk score providers. Machine learning expands the model learning process, allowing more accuracy and consistency in results as new data is added to credit risk routines.
For a deeper dive into how to use Altair’s data analytics and financial services software to mitigate fraud, check out the “Guide to Using Data Analytics to Prevent Financial Fraud” eGuide.
Credit Risk Analytics in Action
At Altair, we provide a myriad of comprehensive solutions organizations can use to optimize their credit risk analytics strategy. For example, lenders need tools they can employ during the loan application process to advance an application to the next step or divert potential customers to alternative products that are a better fit.
Implementing artificial intelligence (AI) models that facilitate fast credit reviews — and even approvals — can help lenders increase the quality, number, and amount of loans they grant without taking on unacceptable levels of risk. Altair helped Fair for You (FFY), a non-bank financial institution that provides loans to low-income families that traditionally have trouble accessing mainstream credit to implement these financial analytics tools.
FFY would like to accept as many requests for credit as possible. However, the cost of lending becomes prohibitive when considering customers who don’t intend to pay back their loans or customers who can’t afford to pay. So FFY’s credit risk team needed to be confident regarding each customer’s default likelihood based on improved insight into the vast amounts of historical data available to them. They sought to implement a real-time scoring system to predict default rates without slowing down the application process. They also wanted to reduce the personnel time required to review individual loans and client credit histories. Automating the application and approval process would help scale their volume without increasing operational costs.
Altair helped FFY develop a scorecard using the team’s existing data. Dividing applications into ten risk bins helped the organization develop specific credit strategies and flexible acceptance rates based on predicted default rates. Once the scoring system was in place, the team formalized a risk band strategy based on default rates and projected total cost of finance.
FFY reduced the initial auto-acceptance and decline strategy to increase the referral bands. Being able to monitor loan applications and default rates by risk band was crucial to the team and the Altair team set up a monitoring dashboard to provide live updates – including key financial statistics with data visualizations – to deliver quick comprehension of trends. The dashboard displays the status of each month’s application and acceptance rates, as well as the current scoring model’s stability, with auto-generated notifications that indicates when the model needs adjustments.
After FFY introduced the Altair-powered scoring solution, its loan acceptance rate increased from 20% to 27% within 120 days, and the number of customers who went into arrears within the first two months of their loan fell by 28%. From the third month onward, the existing arrears rate average aligned with the team’s business strategy and increased acceptance rates among applicants with similar risk levels. Under the organization’s old scoring system, 22% of loans were considered “bad.” With the new Altair-based scoring system in place, only 14% of loans were considered “bad,” which freed up capital for additional loans to more deserving households. Lastly, FFY reduced rejections by 10% and could accept more loan applicants without increasing the organization’s overall risk profile.
Click here to read the full case study, “Real-Time Credit Scoring: Reduce Approval Times, Increase Loan Numbers, Improve Borrower Experience.”
Click on the following links to learn more about Altair’s data analytics and AI tools, machine learning tools, financial services capabilities, and more.