Technological Friction: Examining Data and AI Friction Part Two
Technology is all around us, shaping the contours of our lives and the way we go about work, recreation, and more. The latest battery technology is fueling the electric vehicle (EV) revolution, the advanced chip technology in our phones makes these devices increasingly valuable and powerful, and advances in medical technology and research is helping more people live longer, healthier lives. No matter the industry, technology and the way we use it defines progress.
Today, technological progress is increasingly driven by the latest generation of computational science; this includes things like artificial intelligence (AI), high-performance computing (HPC), simulation, data analytics, and more. As these technologies have grown bigger and more intuitive, organizations are striving to implement organizational data and AI strategies that will help them unlock new levels of productivity, speed, and cost efficiency. But as we mentioned in this series’ first article, these strategies too often run into friction – be it organizational, technological, or financial – that slows down their implementation or cancels it altogether. This article will dial in on technological friction – where it stems from, why it’s occurring, and what impact it’s having on modern organizations.
Prevalence and Causes of Technological Friction
Put simply, technological friction is friction that that stems from technology infrastructure — this includes hardware and software resources, cloud and HPC resources, appliances and plugins, vendors, and more. Often, technological friction acts as a bottleneck by limiting projects’ speed, scale, and/or scope. It’s no secret that the astonishing advances made in computational science require significantly more powerful technological resources than in the past. In our global survey of 2,037 professionals, we examined how this new generation of technology is impacting organizations’ data and AI strategies.
First, let’s establish the prevalence of friction today. According to our survey, friction within both data and AI projects is troublingly common. On the AI side, 42% of respondents said their organization has experienced an AI project failure within the past two years. Additionally, 26% said more than half of their AI projects have failed within the past two years. Within that two-year timeframe on the data side, 33% of respondents said more than half of their data science projects never made it to production; 67% said more than a quarter of their data projects never made it to production. These frequent project failures – often so severe the projects don’t make it to the production stage – hurts organizational morale, eats up valuable employee time, and can negatively impact organizations’ bottom lines.
What did the survey data reveal about the causes of this technological friction? Primarily, respondents struggled most with data processing speed, making informed decisions quickly, and data quality issues. Many of these issues stem from outdated legacy technology that can’t handle the demands and power of modern data and AI tools and strategies. The data showed that legacy systems’ inability to develop advanced AI and machine learning initiatives (33%) and technical teams’ difficulty communicating their needs to other teams in layman’s terms (31%) were respondents’ most common sources of technological friction. Additionally, respondents cited vendor system and software incompatibility as a recurring issue.
Below, you can see the most common challenges organizations face when trying to leverage their investments in organizational data and AI strategies. The data shows that large volumes of messy data derail organizations’ ability to implement successful data and AI strategies.
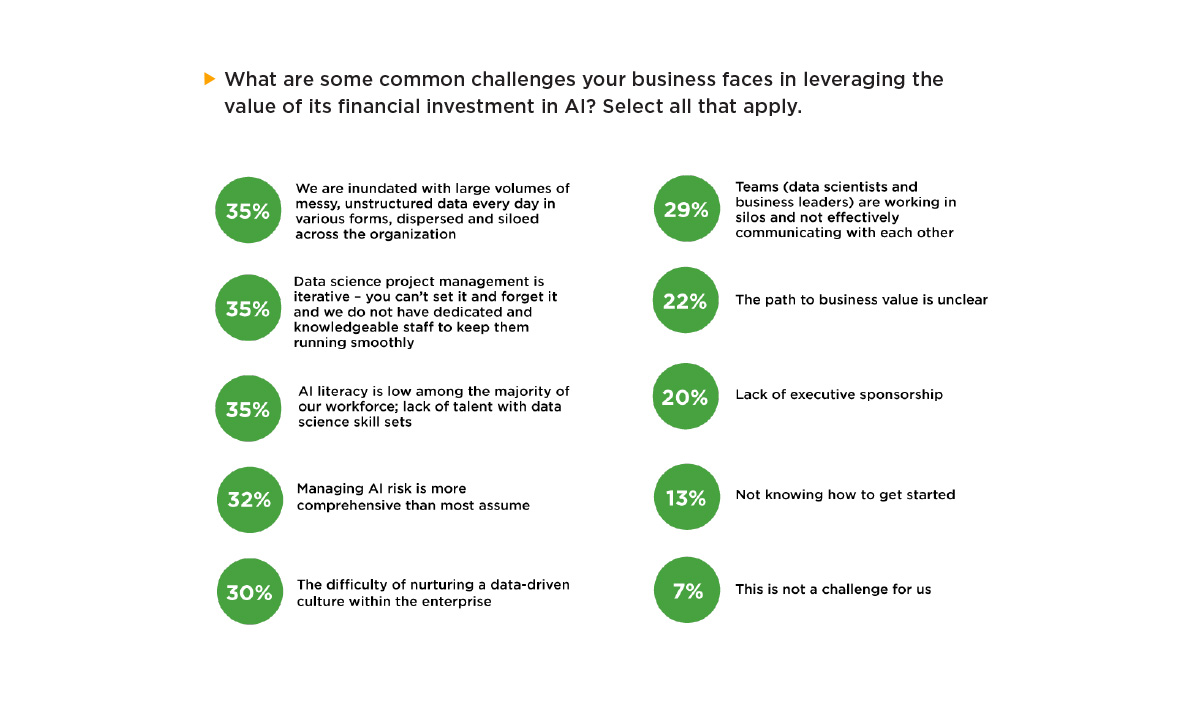
And lastly, the top three issues that prevent respondents from gathering insights from their data are a lack of processing capacity (either in personnel or technology), trouble making informed decisions quickly, and data quality issues.

Conclusion
Technology has come a long way and continues to make incredible strides. That said, as data and AI strategies evolve, the technology needed to execute them needs to evolve too. For many organizations, being stuck with outdated legacy infrastructure has made it difficult to gather, organize, and act on the massive amounts of data we’re now able to collect. In addition, the growing computing needs of AI are putting strain on older compute software and require modern HPC tools to run smoothly and effectively.
As the data from the global survey shows, technological friction is very prevalent and is affecting organizations no matter what industry they’re in. It hampers organizations’ ability to capitalize on the current generation of game-changing data and AI tools, ultimately hamstringing them in the long run. To avoid technological friction, companies must invest in their technology infrastructure so they can reap the long-term benefits of a robust, organization-wide data and AI strategy. Alongside that, they must train and hire knowledgeable people who can operate and coordinate these strategies so they can avoid organizational friction.
Click here to read the next part in this series, "Financial Friction: Examining Data and AI Friction Part Three." To learn more about organizational friction, check out this series’ previous article.
Additionally, be sure to check out more of Altair’s Frictionless AI resources, including:
- Report: Altair 2023 Frictionless AI Global Survey Report
- Webpage: Frictionless AI
- Article: Organizational Friction - Examining Data and AI Friction Part One
- Article: Financial Friction - Examining Data and AI Friction Part Three
- Infographic: AI's Breakdown Lanes - The Three Key Areas of Friction
- Infographic: Why Are AI and Data Projects Coming Up Short?
- Infographic: Achieving Frictionless AI - When, Where, and How