The Symbiosis of AI and Simulation
Today, organizations of all shapes and sizes around the world are facing the challenge of adopting complex simulation and data analysis methods on a broad scale. Through innovative computation methods, user-guided workflows, and know-how transfer, Altair helps companies exploit the benefits of simulation and data analytics, enabling anyone – from complete novice to domain expert – to leverage the true value of data.
The Benefits of AI-Powered Engineering
Wherever the topic of artificial intelligence (AI) comes up, it’s full of buzzwords such as machine learning, generative AI (genAI), and synthetic data. However, these terms say nothing about the benefits of AI. What’s important to know is that AI adoption plays a critical role in modern product development. Especially in the interaction between AI and simulation, new applications are emerging that accelerate processes and better recognize correlations.
While the focus on of AI in engineering is often on algorithm development for data science, the real challenge is identifying value-added use cases and collecting necessary data. The decision to explore AI benefits is largely led from the top, but its success relies on establishing a good policy for data collection, handling, cleaning, and access across traditional silos: including inside the factory, with appropriate suppliers, subcontractors, and through into product warranty and repairs. Likewise, setting up realistic training models to attain insightful results in using accumulated data rather than concentrating on having the most accurate model.
Organizations using traditional metal and plastics manufacturing processes in the design and production components are now investigating the benefits of AI to get ahead of the competition. They tend to share the same concerns of how to get started and the skill sets needed, especially because AI isn’t an “out of the box” solution. It needs nourishing with good data and well-supervised models. Here, we introduce an approach for AI-powered engineering for a manufacturing process, then take a deeper dive into the bespoke megacasting workflow to illustrate the symbiosis of AI and simulation. In this example, AI has been used to easily identify the best designs out of an enormous amount of simulation data by applying machine learning clustering, known as AI-driven expert emulation.
Efficient Model Creation
Based on geometries (mesh or CAD format), algorithms can translate these into values so that the geometries can be compared, edited, clustered, and divided into groups and classes. This makes model organization easier and makes the modeling process more efficient.
Multidisciplinary Design Exploration
With existing results of parameterized designs, regression analyses can be used to identify correlations and make predictions of individual values or behavioral curves. This allows gaps in test data to be filled.
Quickly Predict Physical Behavior
Based on simulated results and geometric structures, a neural network is trained to make behavioral predictions without a new simulation run.
Efficiently Capture Complex System Behavior Using Neural Networks Instead of Co-Simulation
Complex processes that are mapped using computationally intensive simulations, such as the discrete element method (DEM), computational fluid dynamics (CFD), and finite element analysis (FEA), train a neural network that reproduces the system behavior as a reduced-order model (ROM). This makes system simulations significantly faster – in some cases, up to 1,000x faster – without compromising accuracy. This creates opportunities for innovative ideas and system performance optimization.
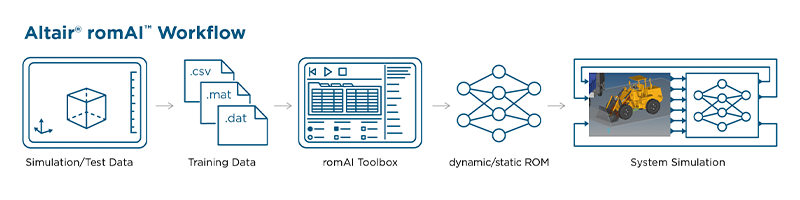
Identify and Optimize Behavioral Patterns
Based on the results of many simulated design variants, an unsupervised machine learning approach is used to create a group with uniform behavioral patterns. This allows hundreds of simulations to be processed intuitively. Here, a recent example of megacasting demonstrates the advantage of the AI-powered approach over traditional methods.
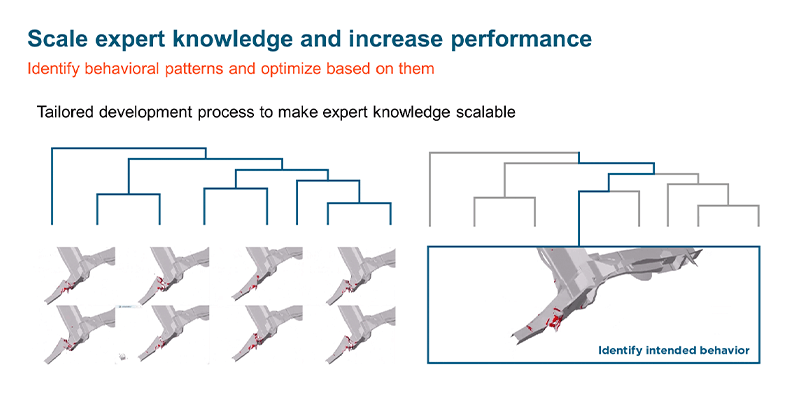
Generative AI Design for Megacasting
The interest in megacasting is widespread, but also presents many unsolved risks. The implementation of large castings requires very large diecasting presses and a high level of precision to comply with performance specifications, production tolerances, and component quality. While genAI offers a new freedom of design for potential lightweight construction, the increasing complexity of producing large, complex geometry castings must be mastered.
Consequently, it’s becoming increasingly important for designers to apply new technologies like AI and machine learning to their approaches for reducing weight, lowering manufacturing costs, and meeting performance requirements.
One solution is a development process that combines data science with engineering. Altair’s AI-powered generative design workflow combines AI, generative design, and the latest CAE technologies. This proven approach enables multidisciplinary variant analyses for efficient, production-oriented megacasting structures. It enables development teams to use thousands of simulations simultaneously to find the best solution. To avoid having to evaluate each design variant individually, the solution space can be restricted to a desired behavioral pattern, meaning that the desired performance can be optimized instead of individual target values. AI-supported generative design helps master the relationship between manufacturing-dependent material quality and component behavior. It allows the most complex components with a wide range of requirements to be efficiently designed and manufactured. To this end, Altair developed a bespoke workflow process that makes expert knowledge scalable.
A Comprehensive Optimization Workflow for AI-Supported Generative Design
In any development process, numerous requirements must be considered to harmonize lightweight construction, functional requirements, and manufacturability.
The optimization process for megacasting components comprises two phases. Starting with topology optimization for efficient material placement based on linearized load cases. This is combined with multidisciplinary optimization to evaluate the component structural performance and check its manufacturability using casting simulation supported by AI and machine learning.
Topology Optimization
Altair’s powerful, proven generative design technique is used to place material most effectively. Here, optimal load paths can be derived for multidisciplinary loading conditions that include hundreds of load cases and variables as well as manufacturing constraints for castings.
Multidisciplinary Optimization
In the aforementioned step, the application of response surface modeling (RSM) optimization is combined with machine learning to meet requirements and provide optimal rib orientations and thickness distributions for megacast structures in nonlinear crash and casting simulations. With clustering and classification of complete simulation result fields, purely scalar, regression-type objectives can be overcome and compared with expert evaluations to optimize for the desired component behavior.
Manufacturability Analysis
Using unsupervised machine learning also enables an evaluation of manufacturability. It recognizes uniform behavior in variants such as the flow velocity at the gate or the flow fronts in the simulation of the die casting process, e.g. to determine the optimal number, size, and position of gate geometries.
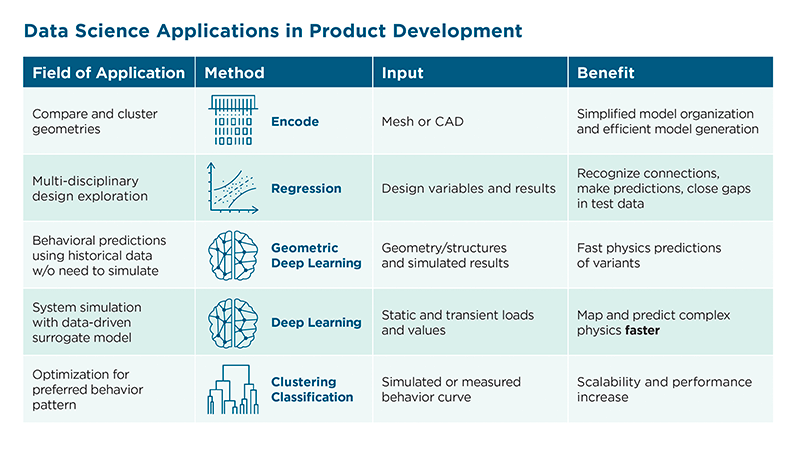
Altair Speaks Both Engineering and AI
With innovative simulation methods, user-friendly, process-driven user interfaces and know-how transfer, Altair helps companies democratize simulation, AI, and data analytics, making it accessible and understandable for professionals and newcomers, reducing the need for physical prototypes.
As a leading provider of data analytics and AI solutions in engineering, Altair's is unique because it speaks both languages: engineering and AI. Altair's no-code AI solutions gives newcomers and experts easy access to machine learning and AI. In all, Altair enables any company to reach simulation and data-driven transformation.
To learn more about Altair’s AI-powered engineering capabilities, visit https://altair.com/ai-powered-engineering. To learn more about Altair’s megacasting capabilities, visit https://web.altair.com/megacasting.