Machine Learning and Artificial Intelligence Inform EV Adoption Levels
One of the largest challenges with managing large datasets is ensuring they are complete. Many use cases can use machine learning (ML) and artificial intelligence (AI) algorithms to accurately identify and fill gaps of unknown historical data sets with data extrapolated from other data sources. We recently put this to the test by analyzing electric vehicle (EV) adoption levels in the U.S. and identifying which states and regions have (or will have) high and low EV adoption. See how Altair’s data analytics solutions rose to the challenge.
Learn how to apply AI and ML to your business.
Contact Us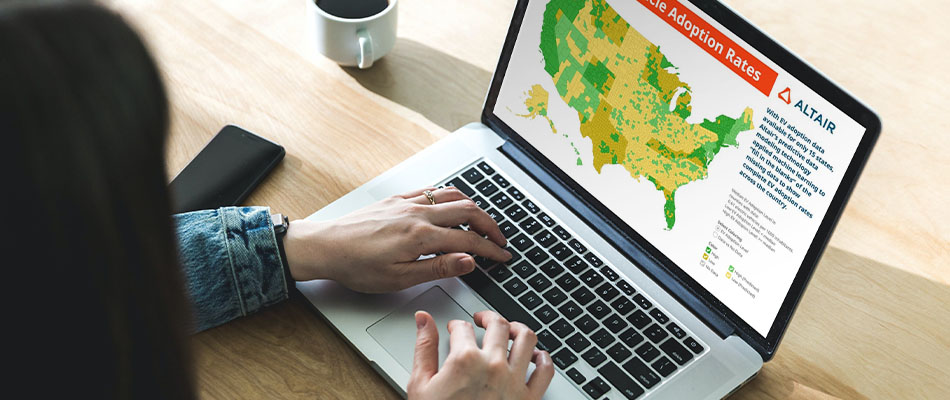
Electric Vehicle Adoption
With EV adoption data available for only 15 states, Altair’s predictive data modeling technology applied machine learning to impute the missing data to show complete EV adoption levels across the U.S.
Data Analytics in Action
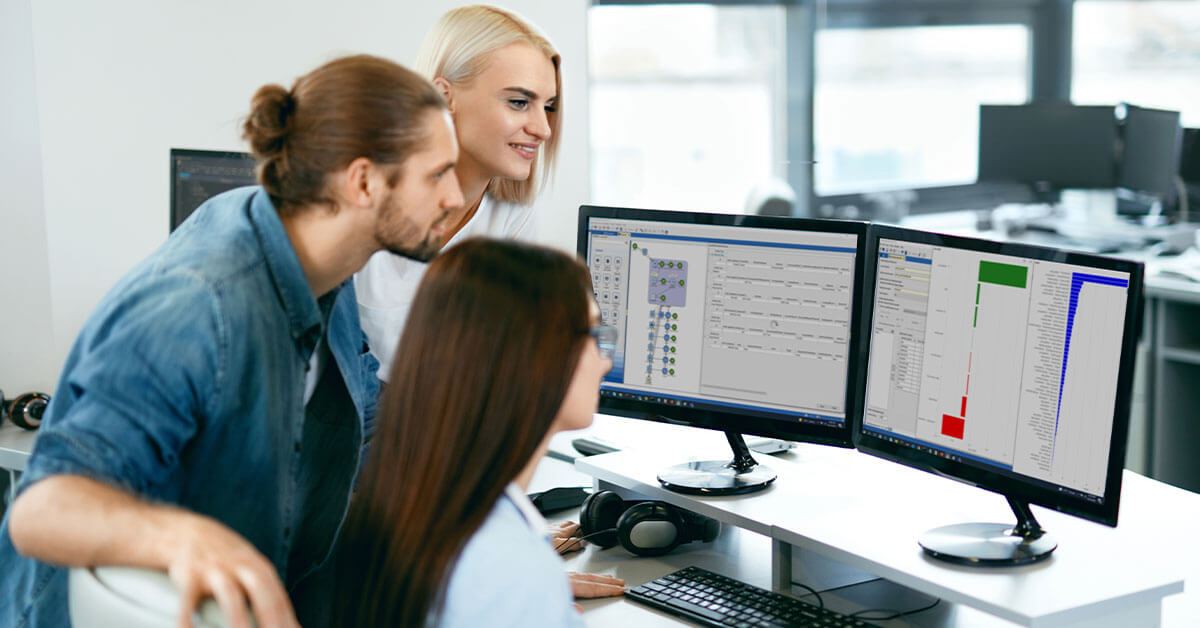
Using Machine Learning to Fill Gaps in Large Datasets
One of the essential problems involved in managing large datasets is ensuring they're complete. Many use cases, including materials databases, can use machine learning (ML) and artificial intelligence (AI) algorithms to accurately identify and fill gaps with data extrapolated from other data in the set. The datasets might contain time series data which, for example, may track the movement of components through a supply chain and/or static data like a parts inventory or test results. Altair's data science tools are well suited to this task.
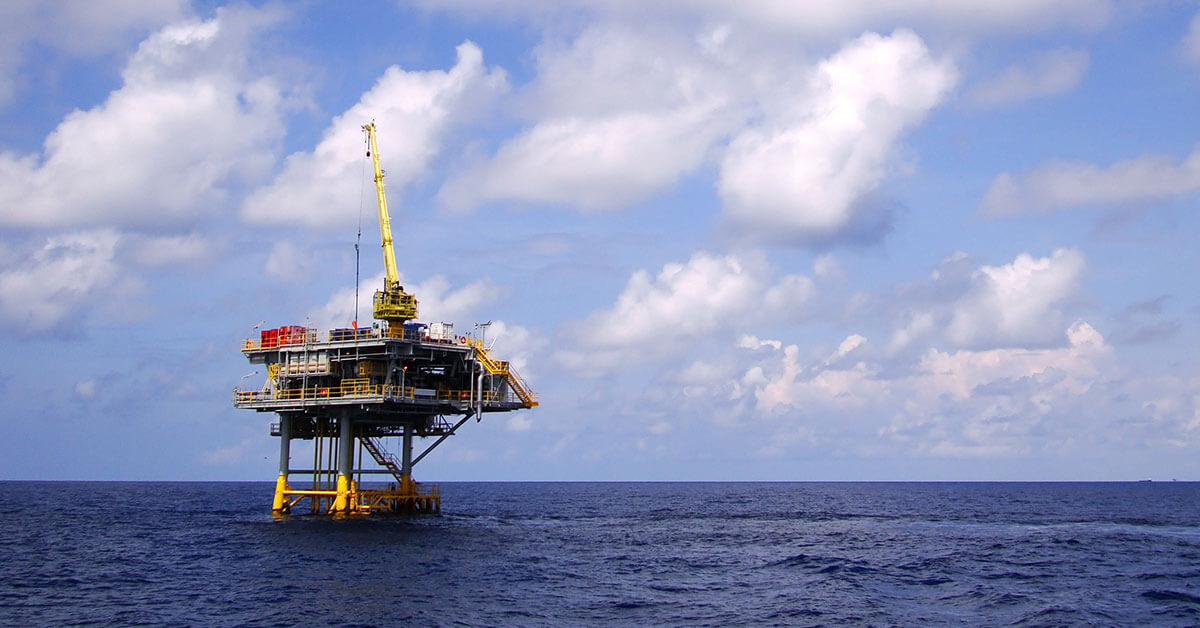
Analytics for Heavy Equipment
Serba Dinamik is an engineering company specializing in operations and maintenance (O&M), engineering, procurement, construction and commissioning (EPCC), and IT solutions for energy exploration and production firms. Their team worked with Altair to develop a Smart Predictive Maintenance Data System (SPMDS) utilizing Knowledge Studio and Panopticon. Maintenance crews use Panopticon-powered dashboards built into SPMDS to monitor every sensor mounted on operating turbines in real time. AI models built with Knowledge Studio identify potential failures or issues that require engineering attention, and, based on that understanding, take turbines offline only when necessary.
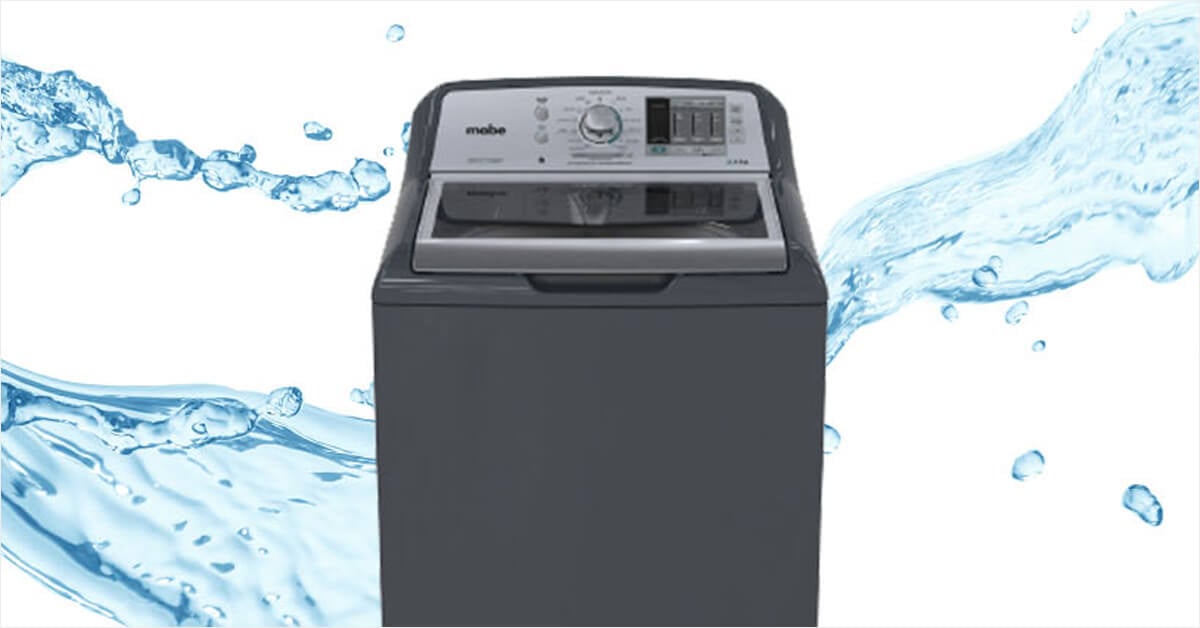
Harnessing the Power of Big Data, AI and Simulation to Accelerate Product Innovation
In a world where everything is becoming more and more connected, Mabe, a leader in home appliances, is leveraging the convergence of big data, analytics and simulation to accelerate innovation. Martin Ortega, Senior Design Engineer at Mabe, explains how they are using Altair's AI, data analytics and simulation solutions to uncover insights, create new business opportunities, and advance product development. Learn more - click here to read how connected products deliver big ROI.

Visualize Power Flows in Real Time
The Electric Storage Company is a Northern Ireland-based firm that manages electric power in households from renewable sources using battery storage and Internet of Things (IoT) technologies. The company installs smart batteries in homes and communities, along with sophisticated management software that lets homeowners sell excess energy back to grid operators when prices are high and helps them maintain the lowest possible energy input costs.
Managing varieties of base load and intermittent renewable power sources requires the ability to ingest, process, and analyze high frequency information emanating from the grid and thousands of devices. The company needs real-time insight into energy markets, the grid, battery systems, and generation facilities, as well as customer-level power consumption patterns. Understanding consumption and generation trends optimizes power routing and battery storage and ensures that power sold back to the grid or on the open market is fetching the best possible price.
Explore More
Data Analytics and AI
Altair enables organizations worldwide to compete more effectively by operationalizing data analytics and AI with secure, governed, and scalable strategies.
Simulation
Use Altair's simulation technology to improve development efficiency, optimize product performance, and accelerate growth.
Internet of Things (IoT)
In a world of smart, connected everything, Altair empowers you to harness the power of the Internet of Things (IoT) to accelerate innovation and unlock business value.