Why AI for Aerospace: Production, Maintenance, and Quality Insights
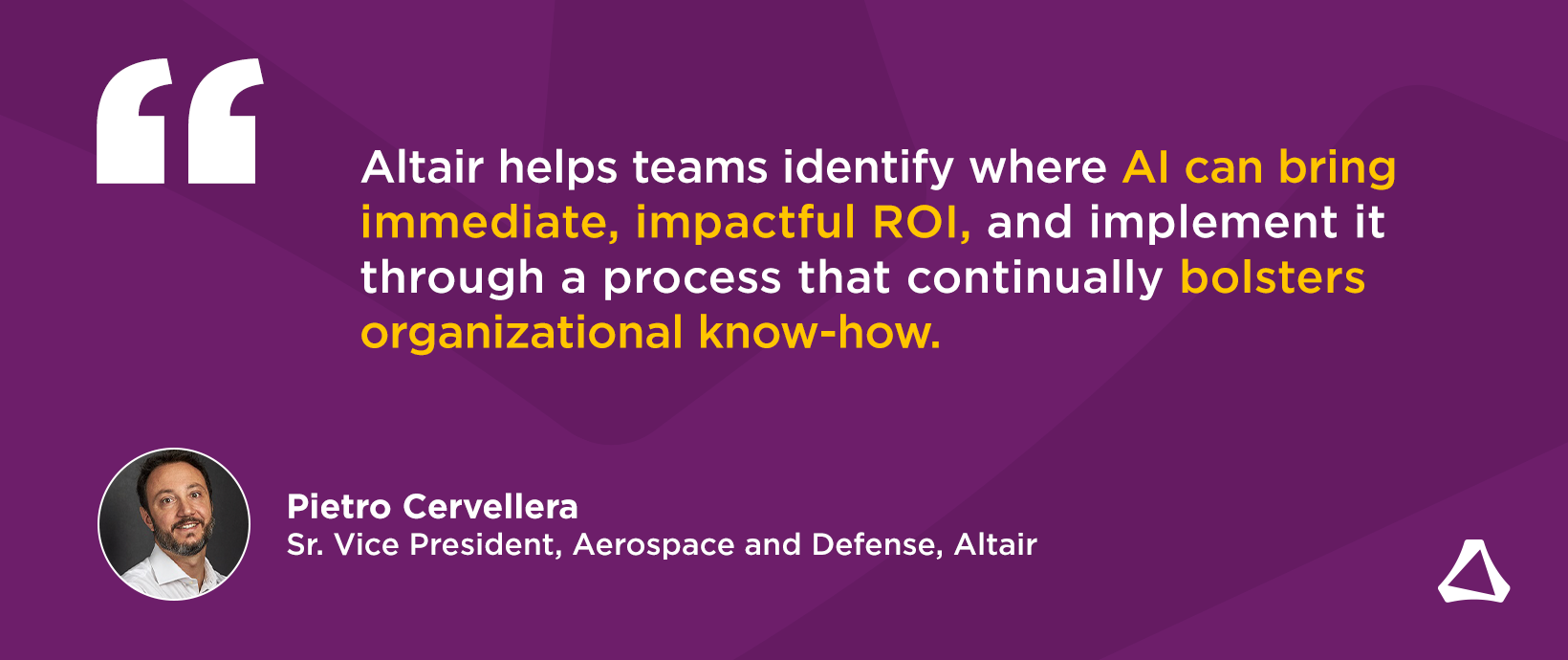
I recently participated in a panel discussion at the 2024 Aviation Forum in Munich, Germany, on how using artificial intelligence (AI) can help maintain quality during production ramp-up. Joined by peers from Airbus, Thales, and Hexagon, we discussed the applications and potential of AI in aircraft production, maintenance, and quality. I would like to share some of my reflections from the discussion, specifically on emerging AI aerospace applications like digital twins.
Where Aerospace Is Using AI
The aerospace industry is facing significant challenges in the way it delivers new components, systems, and aircraft. Order numbers are growing, pushing companies to increase production rates; but in many cases, short-term demand fluctuates, forcing managers to constantly review their production and supply plans. “How do we increase the efficiency of such a complex production system while maintaining quality and safety standards?” is what countless aerospace production professionals are asking. It begs the question: Is AI the solution everyone is waiting for?
One of AI’s main goals in production is to ensure the quality of what is produced internally along with predicting the quality of what is coming from the supply chain. Mistakes here can impact costs and an organization’s reputation. Today, AI in aerospace offers organizations the opportunity to proactively identify defects, predict output quality based on the conditions of machinery, tools, and raw materials, and trigger decisions that affect maintenance or production scheduling, changes in these processes, and beyond.
In fact, organizations are already using machine learning algorithms to turn production machines into smart assets that can make unsupervised decisions and change their working parameters according to data coming not only from the machine itself, but from the entire ecosystem of machines, tools, and the supply chain.
In addition, AI can analyze hundreds of thousands of variables to create a comprehensive picture of how things are progressing on an assembly line. For example, if a part is damaged while installed or if a component needs some reworking, the company can see how this problem (and the sum of many small problems) is impacting the entire production line. This helps managers keep production running efficiently.
In most cases today, AI in aerospace is not replacing workers but rather augmenting human activities. It assists in technical troubleshooting, defect identification, and root cause analysis, with the goal of cutting the time needed to identify a problem and solve it. It can do this very well, up to the point where it can predict and warn an operator in advance that a problem is likely to occur.
Emerging AI Applications for Aerospace
Beyond these uses, there are two very interesting AI applications surging within aerospace. The first is digital twins that support decision-making and scenario analysis. In this case, AI manages data coming both from sensor-equipped assets and from engineering simulation tools—and sometimes from databases, spreadsheets, and previous tests. Altair – a leader in digital twins - can build a dynamic digital twin of an asset; one that is constantly evolving in its ability to predict the behavior of its physical counterpart. These digital twins can be used in the design phase, in which AI can speed analysis by a factor of up to 1,000. Finally, AI often allows users to display information in a way that is more useful for decision-making.
The second is in documentation. The aerospace industry produces a huge amount of documentation. AI is not only helping create documentation, it is also excellent at keeping documentation consistent by identifying mistakes or false correlations. When exploring new ideas, like in the case of startups or revolutionary products, AI in aerospace can make all the difference.
Issues with AI for Aerospace
Despite the endless possibilities AI offers, it also has important limitations to consider.
The first potential issue is data quality. While companies used to mass production invest in modern machines, aerospace companies create fewer products compared to many other industries and are familiar with using old production assets and different generations of machines in the same shop floor. This can make it hard to collect data, and it sometimes requires fitting older machines with sensors, resulting in non-homogeneous signals. As such, a first step to ensuring data quality is being able to collect data from different sources and formats, along with being able to filter this data to manage only those needed for an AI application. This is even a bigger problem if we leave our production line, and if we want to be able to take decisions based on the analysis of data coming from outside sources.
A second problem is the availability of data science and AI talent. A new generation of excellent data scientists is emerging from universities, but there are not enough for everyone. Most of the workforce in service today is still not prepared, so we must find ways to quickly fill knowledge gaps. And beyond people’s skills, AI also has a perception problem; people have a lot of doubts about machine learning models and their results. It is no secret that the concept of AI is still a cause for concern for many people.
Third, a significant gap still exists between the pace of technological change and the pace of regulation. This gap causes uncertainty, and this uncertainty can make companies hesitant to spend time and effort testing new applications that may not be feasible.
Because of these issues, we have seen that most of the companies in the aerospace industry are testing AI – but according to research from Gartner, the rate of successful projects is below 30%. Even successful proofs of concept (POC) sometimes struggle to scale. So, what is hindering success, and how can aerospace companies avoid investing in the wrong projects?
AI Success is a Team Effort
It’s clear within the industry AI must be better understood. When we analyze failed projects, we see many were seen mostly as an IT project done internally by the IT team – who are commonly overwhelmed by requests. Sometimes, the teams working on these projects were even forced to collaborate with external vendors, who impose their own pace. This is all to say that engineers weren’t often very involved. In aerospace AI projects, it's important to collaborate and split the duties between data scientists (who can solve problems) and engineers (who understand the problems in detail and offer domain expertise).
Both teams then have their experts who can understand upfront if there is value in a given application, and where that value lies. If the value is clear—and the people who will benefit from it are involved from the start—the odds of success and scalability become far more favorable.
The Altair Approach
AI and machine learning are not new—Altair should know, since these tools have been embedded in our tools for decades. They speed simulations, automate several “boring” (but important) processes, free up engineering time, and much more.
However, the big change in the last five years has been the convergence of data analytics and simulation to build solutions that can apply to any phase of predesign, engineering, production, supply chain, maintenance, repair and overhaul (MRO), and modernization. Altair’s unique data and AI fabric approach embodies this convergence and gives aerospace companies the tools they need to succeed. It provides an open, flexible portfolio that is easy to learn and use—no specialized data science or domain skills needed—to unleash the power of AI. Our solutions, while still having full-code capabilities, can be used without any coding knowledge, alongside a comprehensive learning hub with certification paths designed for different kinds of professionals.
Summed up, our technology and business model provide companies with a seamless, unified layer for data management, no matter the environment. Our approach also integrates data from different sources and provides a consistent framework for data governance, accessibility, and analytics. Combining usability, a knowledge base, and our expertise on hundreds of different cases, Altair helps teams identify where AI can bring immediate, impactful ROI, and implement it through a process that continually bolsters organizational know-how.
AI is set to overhaul the aerospace industry when implemented with care and foresight—contact Altair today to see how our aerospace solutions can transform your operations and drive unparalleled growth and innovation. To learn more, visit https://altair.com/aerospace.