Artificial Intelligence in Manufacturing: How to Innovate with Speed
One of the challenges manufacturers face today is accelerating design and engineering processes while maintaining accuracy and efficiency throughout the product life cycle. From aerospace and defense to food and beverage production and beyond, it’s common for manufacturers to face high production goals and tight timelines. However, solutions to these problems often require too much money; and navigating budgetary matters while focusing on other needs, such as upskilling, can be difficult. So how can companies optimize output and reach their challenging goals? By improving processes early in the product life cycle by leveraging machine learning and artificial intelligence (AI) in manufacturing.
Why Use AI in Manufacturing Simulation?
Recent advances in AI and machine learning, together with supplementary numerical physics-based simulations, can usher in a new era of product design.
Organizations worldwide are integrating machine learning and AI in manufacturing to create efficiencies and address challenges like reducing downtime and optimizing accuracy, production processes, and decision-making. With them, manufacturers can test different strategies virtually, reducing the need for physical prototypes and minimizing the costs and time associated with trial and error. AI-driven simulations enable real-time adjustment – making designs more responsive to changing demands – and allow users to take these results and validate their final designs.
For example, Altair® physicsAI™ helps manufacturers make physics predictions faster. This tool addresses a critical gap in the initial stages of the manufacturing product life cycle, specifically early when various designs are being considered before there’s a need to rerun simulations. physicsAI leverages the massive amount of synthetic data manufacturers generate; from there, users can input their CAD designs and eliminate the need to conduct new, time-consuming simulations. By leveraging an AI model trained on data from past simulations, users can efficiently obtain precise predictions regarding typical manufacturing processes.
Altair also has various applications Altair® Inspire™, an intuitive, powerful solution that accelerates simulation-driven design throughout the product development life cycle and further integrates AI in manufacturing. When these designs for manufacturing tools are paired with AI-powered solutions like physicsAI, it streamlines and upgrades the entire workflow.
Overcome the Barriers of Entry
A key impediment hindering the broader adoption of AI and machine learning-based simulation is a limited understanding of the advantages it offers compared to traditional simulation. AI- and machine learning-based simulation doesn’t replace traditional simulation – instead, it complements the simulation process by leveraging data organizations already have. Thankfully, these impediments are vanishing as manufacturing leaders continue to embrace machine learning and AI in manufacturing.
AI- and machine learning-powered simulation’s benefits are impactful and far-reaching. For starters, it gives organizations more flexibility. It can be used within many manufacturing process simulations such as injection molding, polyurethane foaming, metal stamping, and casting.
And AI in manufacturing is becoming more common than you may think. For example, with its modern, geometric deep learning capability, physicsAI identifies the relationship between shape and performance for any physics. Once trained, physicsAI models can deliver predictions up to 1,000x faster than traditional solver simulations, enabling teams to evaluate more concepts and make better design decisions. Straightforward workflows let users select trained models, generate predictions, and assess quality for a range of manufacturing processes.
AI in Action
An example of AI improving the early design process is demonstrated in the use case analyzing the porosity of an industrial actuator housing using a high-pressure die casting process. One of the most common problems affecting the functionality of casting components is porosity. Although it’s impossible to achieve zero porosity in the die-casting process, well-planned mold design and process controls can minimize it. In this use case, using Altair® Inspire Cast™ together with physicsAI improved the product design within a much shorter timeframe while maintaining the quality and integrity of the actuator housing unit.
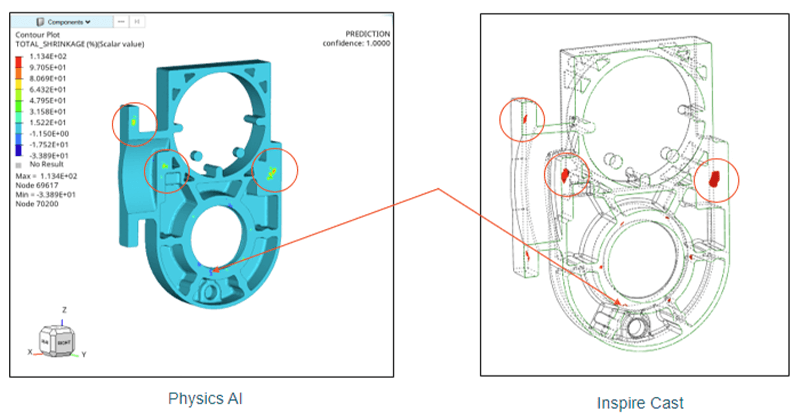
The Importance of AI in Manufacturing
AI in manufacturing is crucial, specifically early in the design process, for optimizing efficiency and precision. For many manufacturing processes, one typically begins with the initial creation of datasets using numerical simulation. With the implementation of AI, once these datasets are determined, users begin training and validating the solver, and using the trained physicsAI model to predict manufacturing defects, reduce design errors and improve the outcome. This structured workflow represents the foundational stages for establishing a methodology integrating Inspire and physicsAI.
Another use case demonstrating the benefits of AI-powered workflows, this time involving Altair® Inspire™ Mold, investigated the warpage of a structural part with ribs using injection molding. Ribs are features added to the primary wall of a plastic part for structural support to resist warpage. In this case, there was a need to run more design models with physicsAI using higher machine learning parameters to achieve a higher degree of accuracy.

physicsAI utilizes the power of users’ CAE data by learning relationships between geometrical shape and full contour results, enabling faster design iterations and generating better designs earlier. Unlike traditional machine learning, physicsAI learns directly from geometry without the need for parameterization. This means users can learn from previous simulations without the need for design of experiments.
Innovate Faster
Manufacturers are creating, gathering, and retaining massive amounts of data throughout the product life cycle. The strategic use of this data enables manufacturers to drive innovation, improve efficiency, and maintain a competitive edge.
In a data-driven world, it’s critical to leverage this information – which is what physicsAI helps organizations do better than any other tool. This tool delivers fast physics predictions by learning from historical simulation data. Although manufacturing processes may vary slightly depending on the product, the logic, and workflow to run physicsAI simulations remain the same.
AI in Action – Again
Another instance of how AI and machine learning accelerates innovation can be found within the metal forming process. For instance, Altair® Inspire™ Form is a complete stamping simulation environment that optimizes designs, simulates robust manufacturing, and reduces material costs. The example below shows a blank size and shape investigated for a typical sheet metal forming process. The size and shape of the blank are crucial for determining the part's formability because they affect the contact surface and friction with the tools. When the test data is more accurate, new design predictions can be made quickly.

Similarly, Altair® Inspire™ PolyFoam predicts and fixes manufacturing problems with rigid and flexible foam product designs earlier in the product life cycle. In another use case showcasing the efficiency of physicsAI and PolyFoam, Altair engineers explored different design variants of a refrigerator door, predicting density distribution and residual stresses. The results confirmed the reliability and importance of an AI- and machine learning-driven approach.

This is another example of how AI- and machine-learning powered simulation solutions allow manufacturers to make better product design decisions early and ultimately deliver production-ready tools on time and on budget.
How Should Companies Begin to Adopt AI in Their Manufacturing Processes?
Manufacturers don’t need to create an FEA model when using AI and machine learning. No matter the application, engineering teams can train a model and within seconds get new design and shape renderings of a particular part and achieve the desired results.
Today, industry leaders around the world are witnessing the positive effect AI has on their manufacturing design processes. For instance, the Delhi, India-based motorcycle and scooter manufacturer Hero MotoCorp Limited – one of the world's largest two-wheeler manufacturers – is reaping the benefits of AI implementation. Jeevesh Prasoon, the company’s senior engineer of digital engineering and testing, explains how leveraging physicsAI has helped their CAE engineers. “physicsAI helps to expedite the output prediction, unlike the traditional FEA approach. It gives an output with roughly 90% confidence matrix and over 99% time reduction and hence enables faster design exploration,” he said. And Hero MotoCorp Limited is but one of many organizations charting a path forward with AI in manufacturing.
Organizations shouldn’t feel intimidated when exploring options for AI in manufacturing. The efficiency and precision AI and machine learning brings to manufacturing processes are valuable. The benefits far outweigh the cost of implementation and optimize product development workflows.
Altair’s simulation-driven design tools are developed with both designers and engineers in mind, ensuring ease of use. And through Altair's patented Altair Units licensing system, users get full access to software tools in the Altair One® Marketplace when and how they need them. More design exploration in less time helps companies discover ways to improve designs early in the development cycle so that they can bring innovations to market faster than the competition.
Explore the links below to learn more about Inspire and physicsAI: